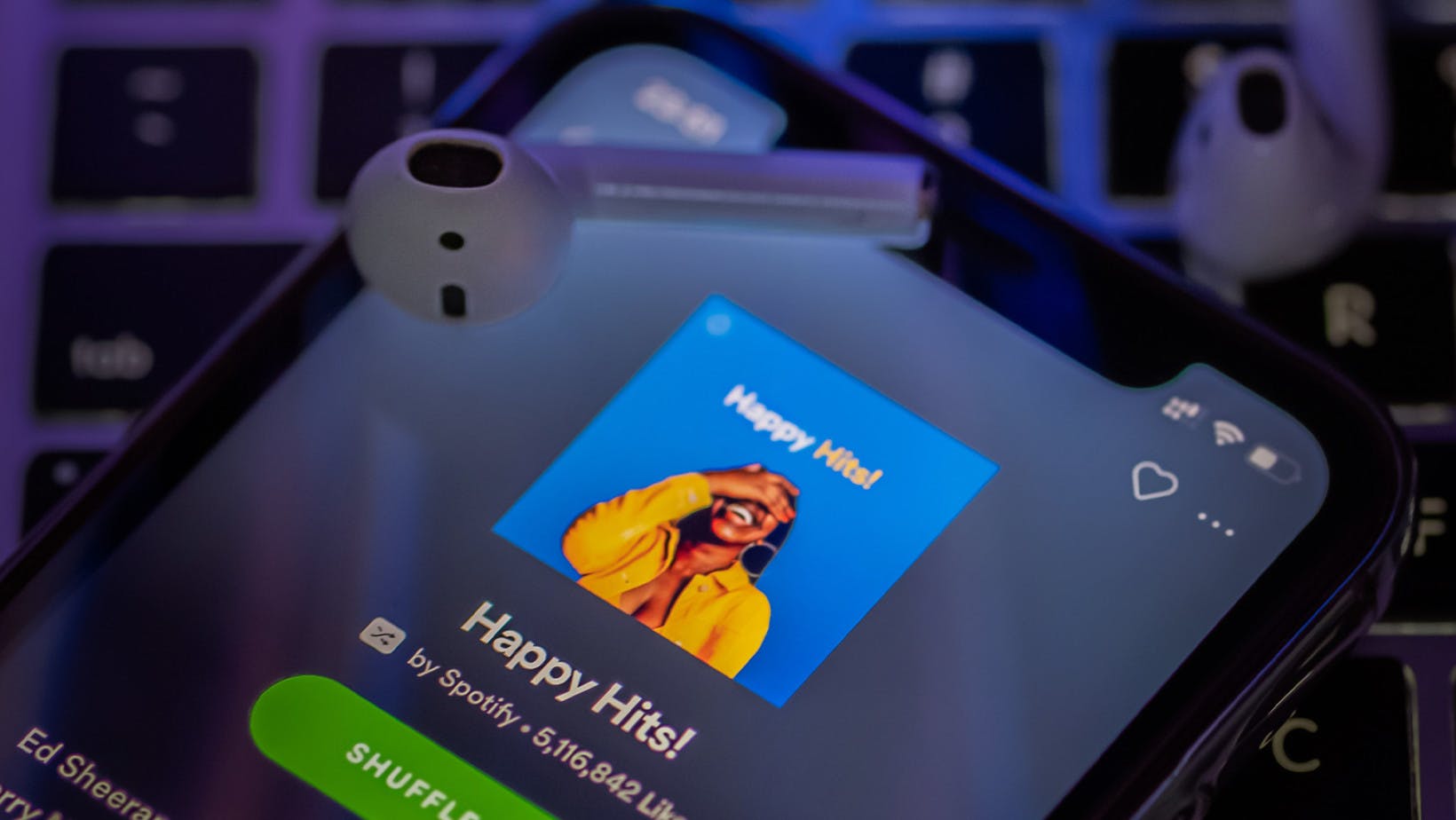
Statistics
What Is Statistical Analysis?
Learn what statistical analysis is and why it’s important. We’ll list the most used types and examples and go over how to do statistical analysis.
Sarah Thomas
Subject Matter Expert
Statistics
02.21.2023 • 7 min read
Subject Matter Expert
Here’s an easy-to-understand overview of ANOVA. We’ll cover why to use it, when to use it , how to use it, and much more.
In This Article
Have you ever wondered how to compare sample averages across many groups in your data set? ANOVA—a statistical method developed by Ronald Fisher in 1918—is a statistical test that allows you to compare means across different sample groups.
In this article, we’ll cover the basics of this method.
Analysis of variance (or ANOVA) is a group of statistical methods used to study the relationship between a categorical variable (or subcategories in your data) and a numerical variable. It is an application of linear regression and one of the methods used in inferential statistics.
Suppose you want to study the relationship between:
Different groups of leading male actors cast in action movies (a categorical variable)
The box office sales of those movies (a numerical variable)
You could use ANOVA to study this relationship.
You may have an in-going hypothesis that movies starring different types of leading men—those with short hair or clean-shaven—on average, have significantly higher box office sales than movies starring other types—like long hair or with facial hair.
ANOVA helps you determine whether these categories do, in fact, explain part of the variation in sales.
ANOVA is useful because it allows you to compare mean outcomes for subgroups in your data. The ANOVA test enables you to determine if a statistically significant difference exists between these group means.
If the difference is statistically significant, you can reject the null hypothesis that each subcategory has the same mean. In other words, you can reject the null hypothesis the categorical variable does not explain any variation in your outcome variable. You reject this null hypothesis for an alternative hypothesis that at least two of the subgroups in your data correlate with the outcome variable.
Where: is the mean for a subgroup
is the number of subgroups defined by your categorical variable
At least two of the group means are not equal;
from some
To use ANOVA, you should identify at least 3 subgroups, and you need to make the following assumptions about your data.
Your independent variable—or explanatory variable—is a categorical variable.
Your dependent variable—or response variable—is a numerical variable.
The population from which observations are drawn should be normally distributed.
Homogeneity of variance exists, meaning the population variance for each subcategory should be the same. We sometimes call this homoscedasticity.
Observations in each of the groups are independent of each other and collected through random sampling. If each observation is independent of others, you will also have independent groups.
When more than two subgroups are in your data, statisticians sometimes prefer ANOVA analysis to performing pairwise T-tests. This is because performing multiple T-tests can increase the likelihood of making Type I errors. However, there are also ways of adjusting the p-values in your T-tests to deal with this issue.
ANOVA has applications in many fields. Whenever a research question revolves around differences between different sub-categories, you should ask yourself whether ANOVA may be useful.
Here are some examples:
Economists studying income inequality may want to know whether parental education levels (high school diploma, some college, college degree, graduate degree) are correlated with the future earnings of children.
A psychologist studying childhood anxiety may want to know whether anxiety levels differ for different age groups.
A business may want to test various advertising campaigns to see whether the ads are linked to improved sales.
A medical researcher can use ANOVA to test whether patients undergoing different types of treatments (Treatment A, Treatment B, Treatment C…) experience different overall outcomes.
Here is a step-by-step example of how to use a one-way ANOVA test.
Let’s return to our hypothetical example of movie stars and box office sales. Let’s say we have data for action films starring lead male actors. We’ll conduct the analysis of variance in three parts.
Use your data to calculate a grand mean and means for each subgroup.
We can first divide the data into four groups based on the leading actor’s hairstyle (bald, buzz cut, short hair, and long hair).
We can then calculate average box office sales for our entire data set. We’ll call this mean the grand mean, .
Next, we calculate average box office sales for each of our four categories .
We might see from our data that the sample means are different, but we’ll need to do a bit more analysis and conduct a hypothesis test to determine whether the differences are due to random factors, such as errors due to sampling, or an actual difference between the groups.
To dig deeper, we start by calculating an estimate of explained variance. The intuition is that if any of our categorical groups do matter, some of the overall variance in our data should be explained by the categorical variable.
Start by calculating the sum of squares total (SST). This is the total of all of the squared differences from the mean.
2. Next, calculate the sum of squares between (SSB). For each group, you’ll multiply the number of observations in the group by the square of the difference between the group mean and the grand mean. Once you’ve done this for each group, you sum up each of your calculations.
Where:
is the number of observations in group
is the mean for group
is the grand mean
3. To calculate the explained variance, divide the sum of squares between (SSB) by the sum of squares total (SST). The explained variance gives you an estimate of how much of the total variance in your data can be explained by the categorical variable.
Suppose you find an explained variance of 0.05 or 5%. This figure tells you that your categorical variable can explain an estimated 5% of your overall variance. The explained variance is also called the effect size.
Just as you would in any type of inferential statistical analysis, you’ll need to test the statistical significance of your results. We can do this by conducting a hypothesis test.
The first step in the ANOVA hypothesis test is to identify the null hypothesis and the alternative hypothesis.
The null hypothesis (H0) is that the population means for each group are not significantly different . This would suggest that hairstyles do not help explain the outcome variable. Our alternative hypothesis () is that at least two of the means are statistically different, which suggests that at least some hairstyles do help explain box office sales.
2. Next, we choose a significance level (or alpha level) for the test. Statisticians typically use an alpha level of 0.10 or 0.05, but the choice is yours to make.
3. To conduct the hypothesis test, you’ll use an F-distribution and an F-test statistic. The F-statistic (sometimes called an F-ratio or the ANOVA coefficient) is a ratio where the numerator is derived from the estimate of variance between the subgroups (the ), and the denominator is derived from the sum of squares error.
Where:
is the F-statistic, or ANOVA coefficient
is the mean square between (also called the model mean square)
# of groups - 1 is the appropriate degrees of freedom for the MSB
is the mean squares within—also called the mean squared error
# of observations - # of groups is the appropriate degrees of freedom for the
is the sum of squares between
is the sum of squares error and is equal to
You can perform all of these ANOVA calculations using statistical software, like SPSS or R, but the intuition here is simple. If the null hypothesis is true, the variance across all of your data (the variance in box office sales across all actor types) should be approximately the same as the variance within each subgroup. The F-statistic will indicate whether the variance across your data is similar or substantially greater than the variance within the groups.
4. As a final step, you can calculate the p-value for your F-statistic. When you perform an ANOVA test using statistical software, you will get an ANOVA table showing you both your F-value and the associated p-value. The larger your sample size, the smaller F-value needs to be for it to be statistically significant.
If the p-value is greater than your significance level, you should fail to reject the null hypothesis. If the p-value is less than the significance level, your results are statistically significant, and you can reject the null hypothesis in favor of the alternative.
As an alternative to this step, you could calculate a critical value and compare your F-value to your critical value. If your F-value is larger than the critical value, you can reject the null hypothesis in favor of the alternative hypothesis.
In these videos, Professor AnnMaria De Mars goes through another ANOVA example looking at psychological distress across groups of patients categorized by their marital status. The first video explains ANOVA, and the second video takes a closer look at the F-statistic.
Some of the limitations of ANOVA are as follows:
The ANOVA test is an omnibus test, meaning it cannot tell you which specific subcategories in your data are statistically significant. To do this, you would need to conduct a post-hoc test, such as a Tukey test, or use other statistical methods.
ANOVA analysis assumes that your data is normally distributed within each group. If this is not the case—if there are outliers or the data is skewed—ANOVA will not give you accurate results.
ANOVA also assumes that the variances are similar across each group. If there are large differences in variance (or standard deviation) between your groups, you should not use ANOVA.
The simplest form of ANOVA is the one-way analysis of variance or single-factor ANOVA test, which we’ve discussed so far in the article. There are other forms of ANOVA tests you could use as well.
Two-way ANOVA. A two-way ANOVA test is an ANOVA analysis that uses two independent categorical variables rather than one.
MANOVA (also called multi-factorial ANOVA or N-Way ANOVA). MANOVA uses multiple categorical variables and tests whether these independent variables are correlated with the numerical dependent variable. The number of independent variables in a MANOVA analysis is three or more.
Outlier (from the co-founder of MasterClass) has brought together some of the world's best instructors, game designers, and filmmakers to create the future of online college.
Check out these related courses:
Statistics
Learn what statistical analysis is and why it’s important. We’ll list the most used types and examples and go over how to do statistical analysis.
Subject Matter Expert
College Success
Learn what statistics is and why it is important to society. We’ll list the types of statistics and data as well as the importance of the sampling methods.
Subject Matter Expert
Statistics
This article gives a quick definition of what’s a residual equation, the best way to read it, and how to use it with proper statistical models.
Subject Matter Expert